April 16th, 2024
Liveness Detection, our Biometric Attack Detection Solution, Is Now Live
We are elevating our biometric identification security with patent-pending 1.5 year-long research outcomes.
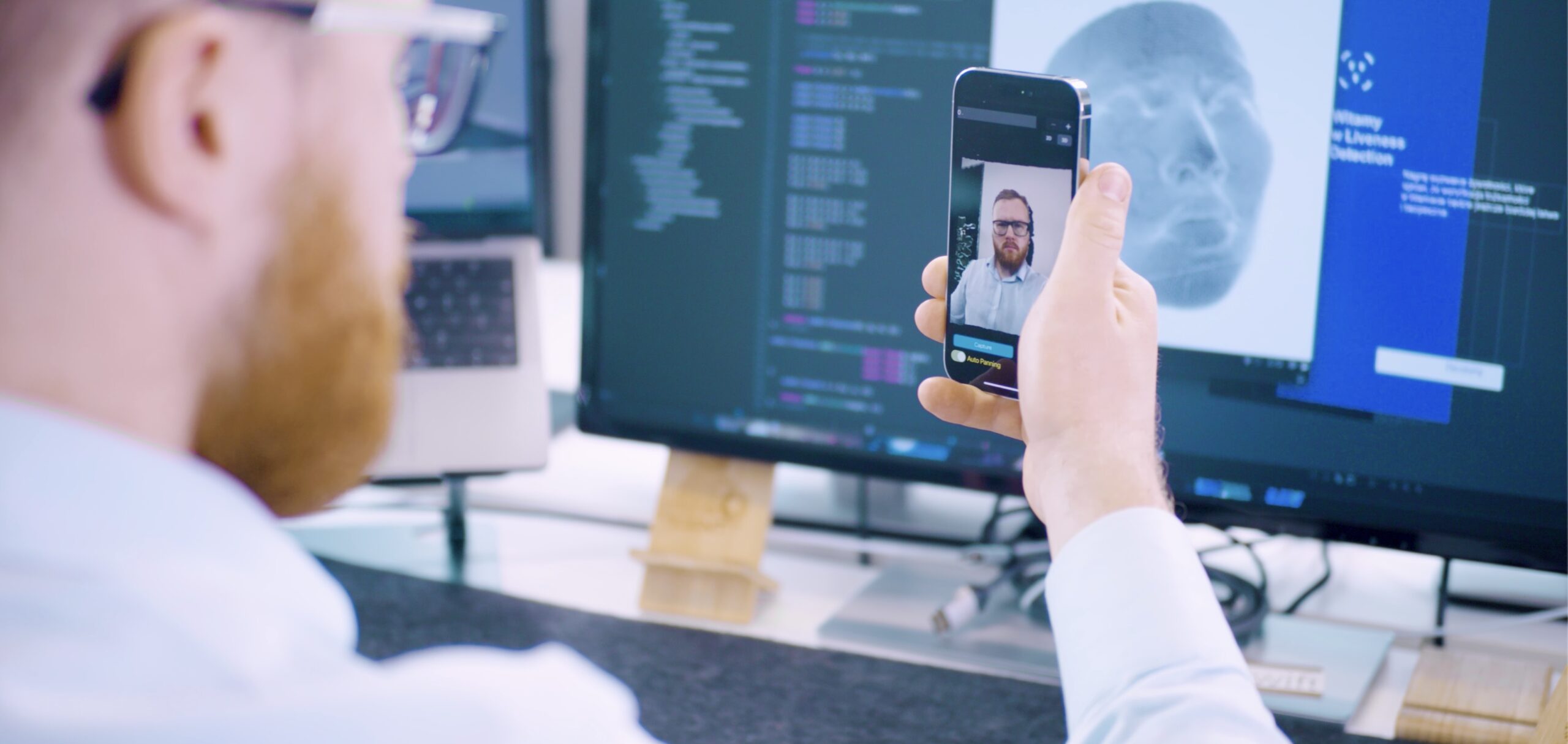
Achieving a significant milestone in our research on biometrics, we are proud to announce a release of Liveness Detection, the state-of-the-art in biometric presentation (PAD) and injection attack detection (IAD).
Towards Unobtrusive Biometric Identification
Based on our 7 years of experience with production-grade biometric systems, Liveness Detection focuses on minimizing user friction while providing an industry-leading level of security expected by our enterprise customers.
To enable this, we introduce new key AI features called:
- Passive,
- Depth, and
- DeepFake Detection.
Combined, these foundational models provide the highest level of security in biometric identification.
In-Background Biometric Feature Analysis
Our new Passive AI automatically captures biometric images during identity verification processes to spot any impersonation attempts.
With no specific user action required, it serves as an unobtrusive guardian, allowing for a faster, more secure, and frictionless identification.
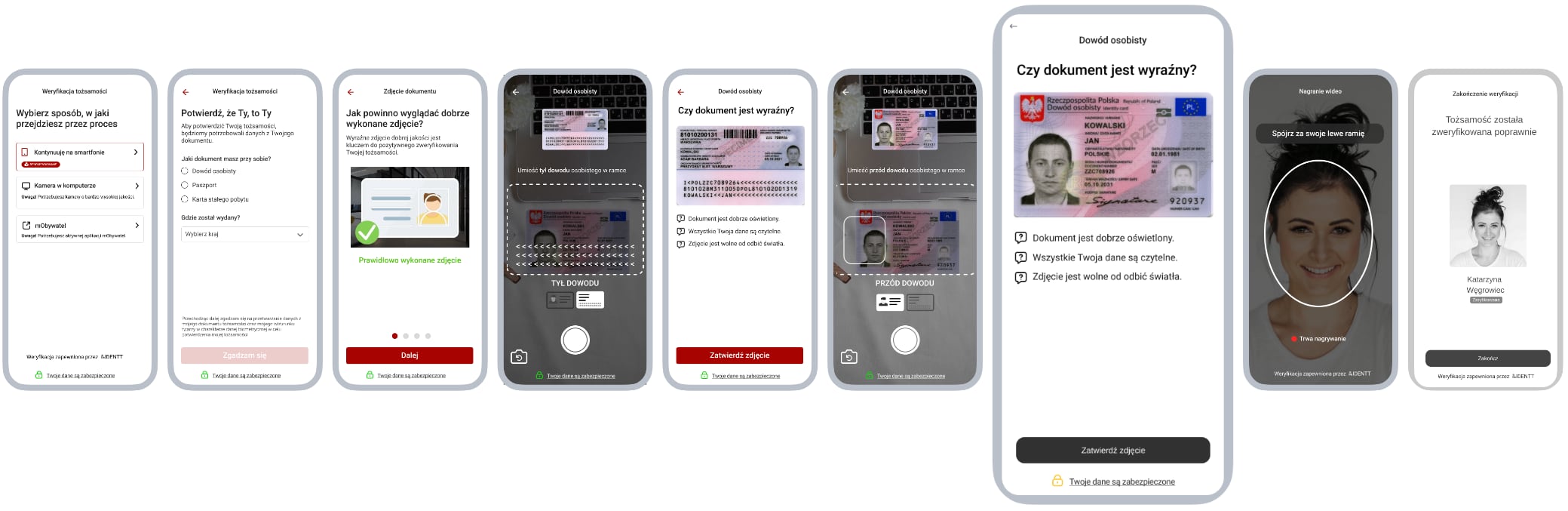
Figure 1. An example identity verification process performed using our Self-Verify web application. During the process, privacy-preserving biometric templates are captured randomly and unobtrusively to ensure the security of identity verification.
Built upon the Vision Transformer (ViT) neural network architecture, Passive AI achieves state-of-the-art results in presentation attack detection (PAD). Tested according to latest ISO/IEC 30107-3 norm. Rigorously tested to ensure the highest level of security across demographic differentials, Passive AI is a proud addition to our family of foundational models.
New Dimension in Identification
With our patent-pending Depth AI, we bring a literal new dimension to face biometrics in identity verification: the 3D.
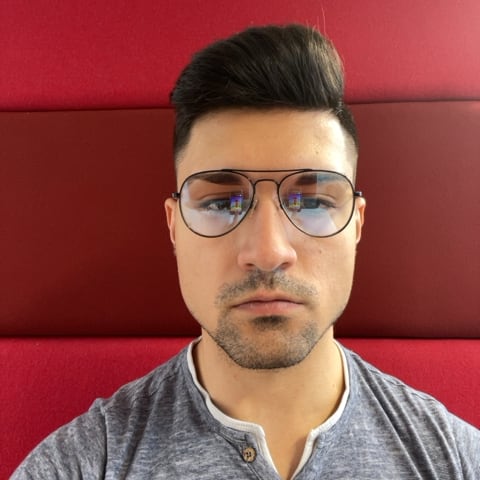
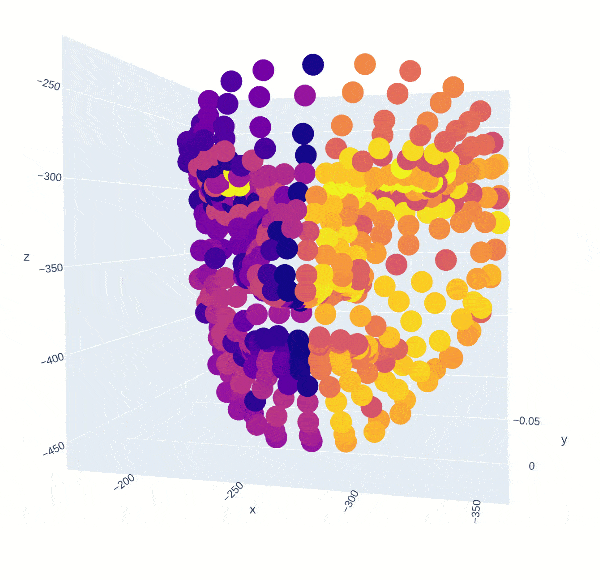
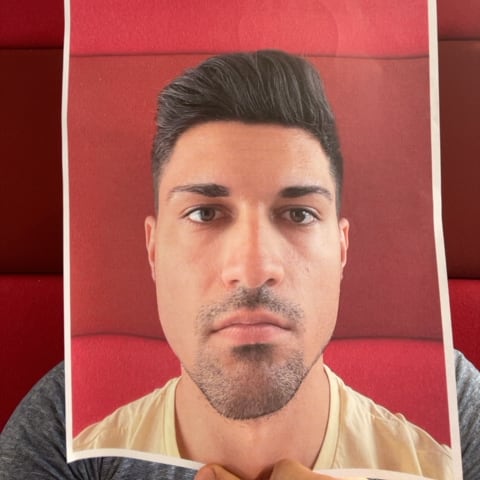
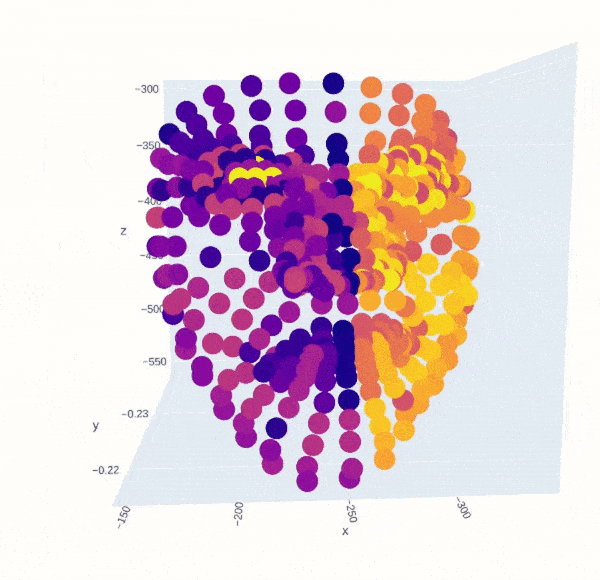
Figure 2. Comparison of Depth AI outputs for bona fide representation (leftmost images) and print attack instrument species (rightmost images). Performing biometric image processing with 3D data allows us to analyze more intrinsic face biometrics, aiding in attack detection and template comparision tasks.
Our solution constructs a time series depth representation of analyzed face. This image helps us detect any spoofing attempts, as well as improve biometric template comparision in mission-critical applications.
Most important of all, no additional sensor required. All it takes for an user is to move the phone closer.
DeepFake Generalization With Unknown-Domain Data
Our goal was to ensure a robust DeepFake detection solution. With DeepFake Detection AI, we have developed a novel neural network architecture and training protocol, improving on SOTA performance for the task.
To achieve this, we used known DeepFake generator outputs for our manually-engineered bona fide dataset aimed specifically at identification use cases. Furthermore, we’ve analyzed known DeepFake attacks generated with unknown sets of tools to improve our ViT-based classification method.
Figure 3. DeepFake generation method output for an input sequence (leftmost video). With known GenAI methods, it becomes increasingly less complex to perform an injection attack on an unguarded biometric system.
Like all of the other components, DeepFake Detection AI is designed to be constantly evolving. New data engineered by our Data Science teams in accordance to the protocols developed during our research ensures fair fight against ever-evolving GenAI methods.
Future Directions
Our foundational models: Passive, Depth, and DeepFake Detection AI are a bedrock for our future innovations in biometric identification and authentication.
With upcoming revision of ISO/IEC 29794-5 norm on biometric template quality assessment, we aim to provide new sets of rules for automatic assessment of input data for our systems, further improving the robustness of biometric identification.
With upcoming UI-assist features both for end users and system operators or explainable AI features, Liveness Detection is set to achieve new standards in accessible security and trustworthiness.
Liveness Detection, the Biometric Attack Detection Solution from IDENTT.
Research and Development Programme Co-Funded by the European Union.
Supported by the European Regional Development Fund under the Smart Growth Operational Programme for 2014-2020. Funds appropriated under the National Center for Research and Development Fast Track (Szybka Ścieżka).
About us
is an artificial intelligence (AI) research unit of IDENTT delivering core automations that enable robust and secure remote identity verification.
Our Artificial Intelligence
helps industry leaders across the world build new, digital onboarding and authentication experiences under mission-critical reliability requirements.
Our Team
consists of top world researchers in biometrics and identity document presentation or injection attack detection, with acclaimed publications and independently recognized expertise.
